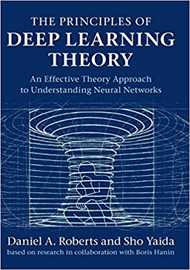
Given the widespread interest in deep learning systems, there is no shortage of books published on the topic. This book stands out in its rather unique approach and rigor. While most other books focus on architecture and a “black box” approach to neural networks, this book attempts to formalize the operation of the network using a heavily mathematical-statistical approach.
The analogy is with thermodynamics, where one can stop at the macroscopic quantities that describe a system (temperature, pressure, volume) or dive into the statistical equations that describe how macroscopic quantities arise from the collective interaction of individual molecules.
The book is a joy and a challenge to read at the same time. The challenge is in plowing through page after page of complex multi-line equations, some with not entirely tongue-in-cheek references to sources that can provide even more math. The joy is in gaining a much deeper understanding of deep learning (pun intended) and in savoring the authors’ subtle humor, with physics undertones.
The book includes an incredibly detailed index and just about 100 references, focused on many classic publications, as well as many recent ones on ArXiv. In a field where research and practice largely overlap, this is an important book for any professional; using it as a textbook might be rather daunting.
Review by Bogdan Hoanca, University of Alaska Anchorage, USA.
The opinions expressed in the book review section are those of the reviewer and do not necessarily reflect those of OPN or its publisher, Optica (formerly OSA).